07 May 2024
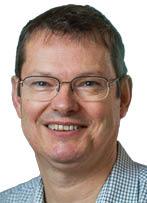
Richard Brandon, VP strategy at RtBrick
Artificial intelligence (AI) is all the talk recently, and adoption into daily work life is something that is taking off globally. The UK has already shown its interest in being a global leader when it comes to AI research, as it hosts the AI Safety Summit in November. In 2023 alone, we’ve seen a 48% increase in searches for various AI platforms in the UK.
Clearly the level of interest in AI has grown drastically recently, however before we get ahead of ourselves with AI innovations, it is important to consider the basics and remember that to achieve the required processing speeds, AI can demand a significant network capacity. For AI to realise its full potential as a valuable tool in the workplace, networks must possess the capability to dynamically adjust to various real-time situations.
Are today’s networks strong enough for AI?
Think about how we currently depend on the capacity of AI to handle and assess vast volumes of data. In the realm of business, AI’s remarkable processing and automation capabilities will transform into an indispensable resource, liberating us from mundane tasks and allowing us to be more imaginative and socially engaged. However, AI, as a technology, is still in its early stages.
As AI advances, its hunger for computing resources will grow. It seems we often assume that our conventional network infrastructure will also cope with this increased demand. However, the truth is that it won’t, unless it undergoes its own evolution.
What is the relationship between AI and networks?
Drawing parallels between AI and networks to how the human brain manages intelligence is a great way to understand the role of networks in AI.
A common mistake is to perceive AI as being ‘smarter than us,’ yet we often overlook the fact that our brains are proficient at processing a similarly impressive network of cognitive, emotional, and dynamic data. Interestingly, our brain’s capabilities are also constrained by the size of our neural networks. Furthermore, another crucial aspect of our intelligence lies in our capacity to receive and process new information from our environment. Our brains continuously contend with vast amounts of intricate sensory data, akin to an ‘Input and Output (I/O) capacity’ when considered in terms of computing.
“AI has the capacity to process and compare virology reports and patient symptoms from anywhere in the world, potentially detecting and tracking potential pandemics before they escalate.”
AI is still relatively nascent as a technology, and as it matures, it will aspire to expand in both dimensions. This will require stronger, scalable networks when we consider time-critical applications.
Presently, a substantial portion of AI applications primarily revolve around learning processes, such as language acquisition, medical symptom-to-cause correlations, or the analysis of financial patterns. These tasks heavily demand ‘processing and storage’ capabilities but are seldom time-sensitive. They are unlikely to strain the input/output capacity, even if the computational and storage resources within the data centre or AI-cloud need to be interconnected to facilitate scalability. So, these current uses of AI are well-suited for the current state of networks.
What does the future of AI look like?
Everything takes a different turn when AI must adapt to real-time scenarios. To unlock AI’s full potential, especially in critical domains like disaster recovery and emergency healthcare, network adaptability is essential.
Consider these scenarios as the potential AI paired with strong networks has to better the world: AI has the capacity to process and compare virology reports and patient symptoms from anywhere in the world, potentially detecting and tracking potential pandemics before they escalate. Similarly, it could analyse seismic data to predict earthquakes or monitor cybersecurity activities, mapping vulnerabilities and forecasting potential attacks. The possibilities of AI are extensive.
What will the future implications of AI development be if we don’t upgrade our networks?
For the situations listed above to become a reality, we demand a greater network capacity than that we currently possess. This means that we must make upgrades to keep up with the formidable computing capabilities of AI innovation.
It’s important to note that we’re not just talking about bolstering the network capacity within data centres but expanding the capacity to individual users and devices at the network’s periphery – essentially, mobile, and broadband networks. However, currently these networks are ill-prepared for the impending growth of AI, and the only viable solution lies in either enhancing these networks or imposing limitations on AI’s progress.
As experts continue to discuss the potential risks and benefits of AI, it remains somewhat ‘up in the air’ until we address the issue of our network capacity. Unless we act promptly, we risk having an incredibly advanced AI that operates far below its true potential, and that would not be smart.